Influencers & Outliers
By Mariska Burger
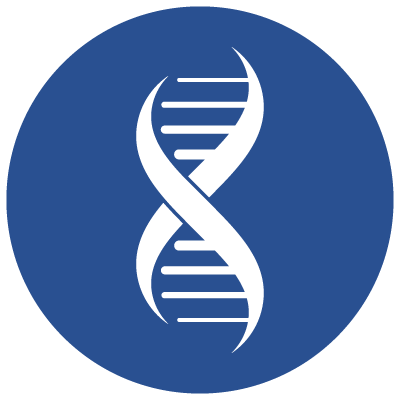
In today’s modern age social influencers are getting paid to post something on social media. In 2020 Christiano Ronaldo was the highest paid Instagram influencer getting paid an average of $466,100 to $777,833 per post. Outliers, spreading fake news on social media are not paid (or are they?) and are definitely not welcomed on social media. In statistical analyses we also have influencers and outliers that can have a significant impact on the fit of a statistical model. When we perform regression analyses, for example, we need to verify that the model is not heavily affected by some individual observations. But are outliers influencers and are influencers outliers? What is the difference and how can we easily identify it?
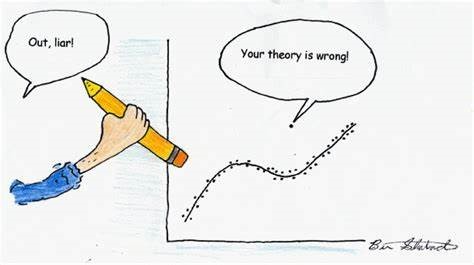
An outlier is defined as an observation that has a big difference between its observed and predicted values, which can be seen with large standard or studentized residuals (Chatterjee & Hadi, 1986). An influential observation, as stated by Belsley et al. (1980, p11), are observations “which, either individually or together with several other observations, have demonstrated larger impact on the calculated values of various estimates…than is the case for most of the other observations. ”Chatterjee & Hadi (1986,section 3) demonstrated that an outlier may not be influential and an influential observation may not be an outlier. Observations with high/low studentized residuals are outliers on the dependent (Y) variable, while observations with high leverage are considered as outliers in the independent (X) variables (Chatterjee & Hadi, 1986). We can visually inspect the studentized residual plots and flag any observation that falls outside the [-2.5, +2.5] interval as potential outliers.
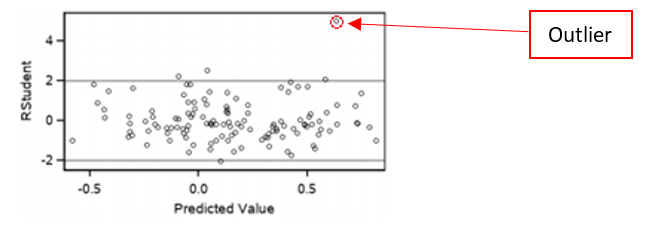
There are several statistics, which can easily be requested by most software packages, that measure the influence of an observation, like for example:
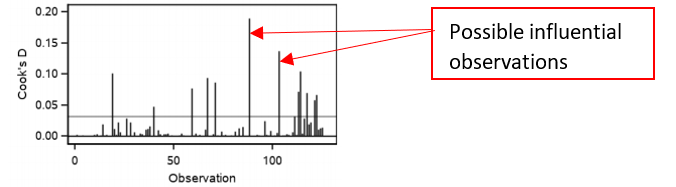
- Cook’s distance - measures the influence of an observation on all fitted values
- DFFits - measures the influence of an observation on its own fitted value
- DFBeta - measures the influence of an observation on a particular regression coefficient
In all cases higher values indicate observations with greater influence.
Should you remove all influential observations or outliers? Outliers or influential observations that arise from human or measurement errors can be excluded from the analyses. The impact of other outliers or influential observations should be investigated by removing them sequentially, starting from the most likely one. Stevens (1984) suggested to conduct the analyses with and without the influential observations or outliers and to report on the impact of the observations to maximize transparency.
The impact of social influencers is huge and most people trust everything these influencers say on social media. However, the impact of influencers in statistics cannot be trusted and should be evaluated. Outliers are frowned upon and definitely not welcomed on social media and also not in statistical analysis. Make sure your statistical model can be trusted, by identifying and evaluating the influencers and outliers.
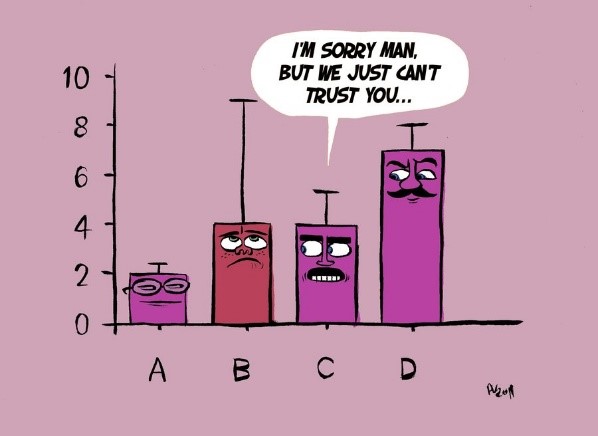
Sources
Belsley D.A., Kuh E. & Welsch R.E. (1980). Regression diagnostics : identifying influential data and sources of collinearity. New York: Wiley.
Chatterjee S. & Hadi A.S. (1986). Influential Observations, High Leverage Points, and Outliers in Linear Regression. Statistical Science 1: 379-393.
Stevens J.P. (1984). Outliers and influential data points in regression analysis. Psychological Bulletin 95:334‑344.
Belsley D.A., Kuh E. & Welsch R.E. (1980). Regression diagnostics : identifying influential data and sources of collinearity. New York: Wiley.
Chatterjee S. & Hadi A.S. (1986). Influential Observations, High Leverage Points, and Outliers in Linear Regression. Statistical Science 1: 379-393.
Stevens J.P. (1984). Outliers and influential data points in regression analysis. Psychological Bulletin 95:334‑344.